How To Spot Misleading Charts: Check the Chart Design
Charts are all around us. When communicating with data, viewing a chart instead of a table of numbers helps us quickly understand data, make comparisons, see patterns, and make better decisions. In today’s world, swift decision-making with data is crucial. When viewing and creating charts, it’s vital that we gain the ability to critically discern the integrity of the information and conclusions shown in charts.
Many people don't realize that charts are as flexible and malleable as the written word. In the same way that words can deceive, so can charts. In a world of increasing misinformation, it is vital everyone has the skills to spot the tricks used by some. It's also possible to accidentally create misleading charts if one has gaps in their data literacy: these pitfalls outline ways to ensure our own charts fit to high standards.
To aid your thoughtful review of charts, we created a handy 4 part checklist with an easy to remember acronym, SCAM. SCAM stands for Source, Chart, Axes, and Message. Be aware of the SCAM! This blog will cover the second part of the checklist—check the chart design. Read on to learn how to spot misleading charts with confidence and continue your learning with the rest of the checklist:
- How to Spot Misleading Charts: Know the Source
- How to Spot Misleading Charts: Check the Axes
- How To Spot Misleading Charts: Review the Message
Learning chart design best practices can help you accurately interpret data. Read on to explore questions to ask and chart design best practices to consider while reviewing charts.
Check the Chart Design.
Learning chart design best practices can help you accurately interpret data. Read on to explore questions to ask and chart design best practices to consider while reviewing charts.
Is the chart the right type for the data being presented?
To understand the right chart type for the data being presented, we need to consider quantitative and qualitative variables.
Quantitative variables can be measured numerically. Examples of quantitative variables are the number of items in a set or height in inches. When displayed on an axis, quantitative variables will have scales with even intervals (0, 5, 10, and so on).
Qualitative variables can’t be measured numerically. Examples of qualitative variables include favorite food, movie genre, or Sales region. When displaying qualitative variables, the axis will show categories instead of scales with even intervals.
To learn in more detail about types of variables, check out the Variables and Field Types module in Trailhead and Tableau’s help article on Data Structure.
There are different chart types appropriate for the type of data being presented. Common chart types appropriate for qualitative variables include bar charts and pie charts. Some common chart types appropriate for quantitative variables include scatterplots, line charts, histograms, box and whisker plots, and bar charts. For a deeper dive into best practices for reading and creating charts, checkout the Communication with Charts module in Trailhead.
Watch out for charts that use types of charts not meant for the type of data being presented. For example, you may encounter line charts, which are meant for showing quantitative data, used with qualitative data.In the inappropriate line chart below, the chart makes it appear that there are even intervals between categories. When we study the axis, we discover categories of sectors (Agriculture, Arts, Clothing, and so on) are not numeric and do not have equal intervals. It does not make any sense to connect them with the line. A bar chart would be the better alternative.
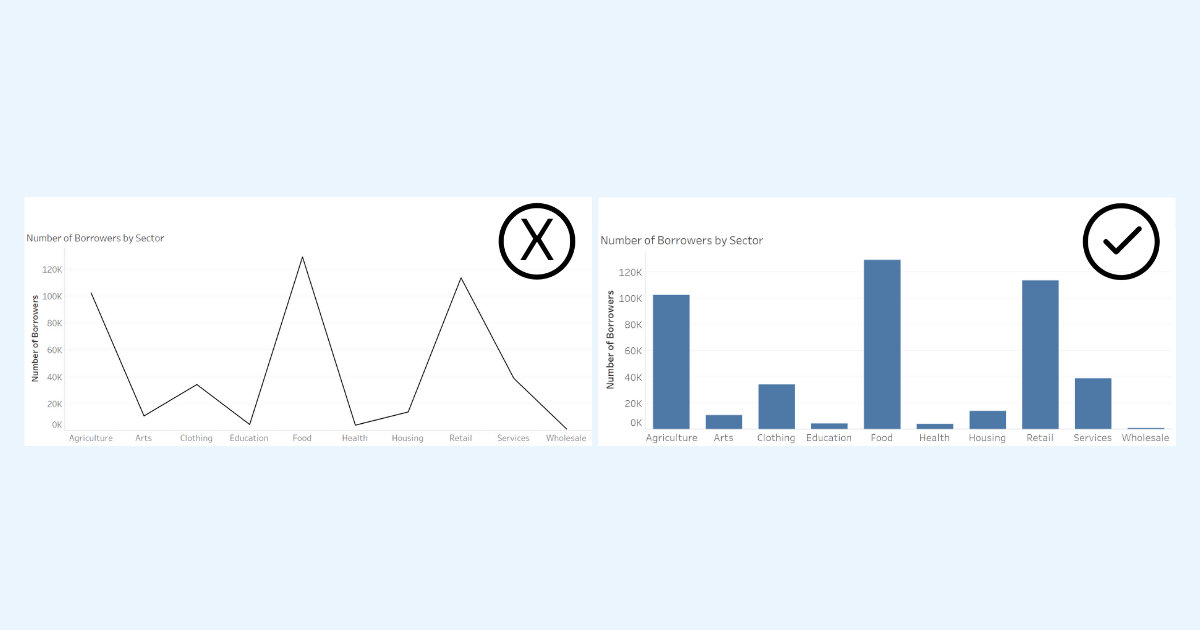
Inappropriate line chart using a qualitative axis (left) and an appropriate alternative bar chart (right).
Are there any misleading design practices used in the chart that might deceive or distract you from correctly interpreting the data?
While creative and intriguing designs can be eye-catching, some can distract from properly communicating important insights in the data.
For example, while pictograms that use symbols and images to convey information may add visual appeal, when they don’t follow best practices, they may mislead viewers—particularly when used to display size differences. This is evident in the pictogram below, where you’re misled into seeing a much larger difference between the two categories than what actually exists.
The underlying data is that A = 100 and B = 300. Looking at the chart, viewers are more likely to interpret the area between A and B in the chart rather than height as the difference between A and B. Using area in this pictogram misleads us into thinking there is a 9-fold difference instead of the correct 3-fold difference. This pictogram violates the Principle of Proportional Ink described by Carl Bergstrom and Javin West at the University of Washington: when a shaded region is used to represent a numerical value, the area of that shaded region should be directly proportional to the corresponding value.
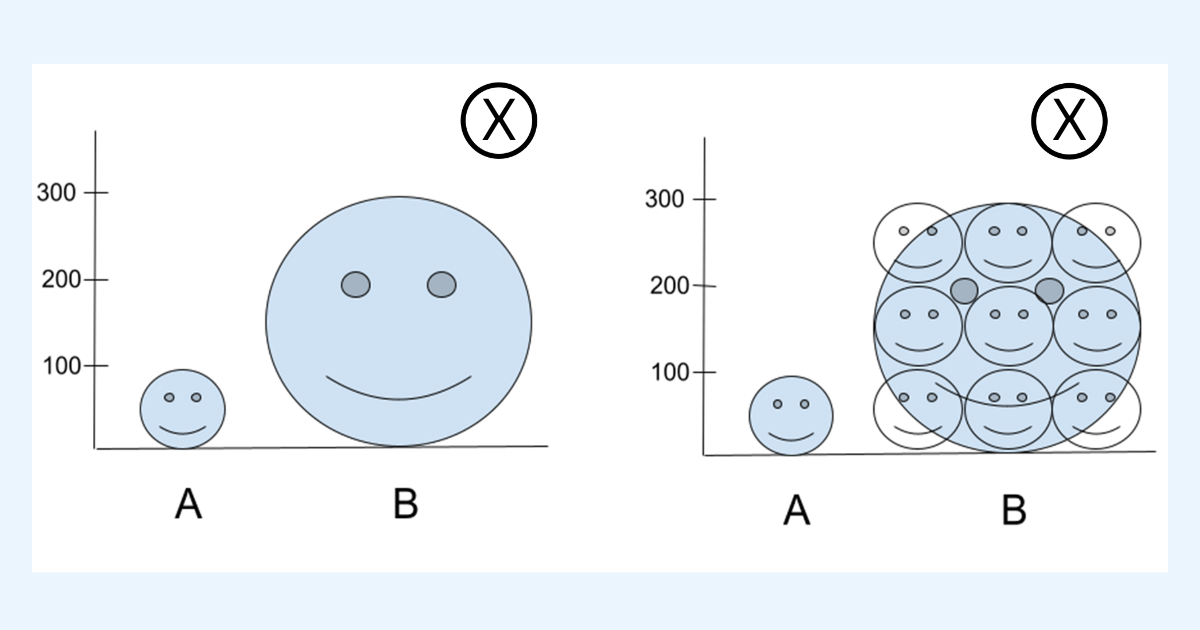
Two pictogram charts: the pictogram on the left showcases two smiley faces, a smaller “A”, and larger “B”. The pictogram on the right showcases the same “A” and “B” but also includes how many smaller “A” smiley faces would make up the larger “B”. While value B is only 3 fold more than A, the larger smiley faces lead the reader to see a 9 fold difference instead.
Take the Avoid Misleading Chart Design unit in the Guidelines to Recognize Misleading Charts Trailhead module where we cover more design practices to watch for.
Practice and be confident
Interpreting charts can be challenging work. You can all help improve how decisions are made every time you accurately read charts or call out misleading charts that you encounter. It’s important to practice. Follow the SCAM checklist every time you see a chart in your daily life.
Share and help the community
While it’s important to think critically and ask yourself smart questions, it can be extremely helpful to analyze data with others. Discuss your interpretations and chart critiques with your peers and friends. You’ll find that you can help improve the decision-making within your organization and help stop misinformation in our communities.
Want more data resources?
Data Skills Resources: Whether you’re a seasoned data veteran, or totally new to the world of data, we have the resources to fill in the gaps, or build a solid foundation to launch from.
Tableau Community Resources: Just getting started, expanding your network, or growing your skills? We’ve got personalized resources and recommendations at the ready to help you reach your goals.
Tableau Academic Resources: Want to use this information in your academic classroom? Visit the Tableau Academic Curriculum website to request access to this information and more.
Guidelines to Recognize Misleading Charts: A five unit badge that will help you accurately read and present chart data and avoid misleading chart designs. A perfect follow-on after reading this blog!
Communication with Charts: A five unit badge that will help you apply best practices for designing and reading charts to tell the story of your data.
Build Your Data Literacy: Seven badges that will help you explore, interpret, and communicate effectively with data. The trail covers topics such as the basics of data literacy, aggregation and granularity, well-structured data, distributions, understanding variation, and correlation and regression.
Alberto Cairo (2020): How Charts Lie: Getting Smarter About Visual Information,W.W. Norton & Company: In-depth guide on how charts can mislead us. Helps you be a critical consumer of data visualizations.
Articles sur des sujets connexes
Abonnez-vous à notre blog
Obtenez les dernières nouvelles de Tableau dans votre boîte de réception.